Text Annotation Usability to Machine Learning
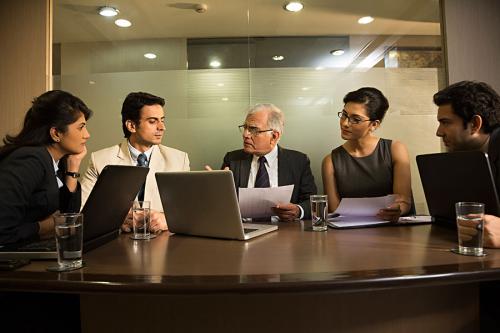
Although the world has shifted rapidly towards digitization, some documents and papers still contain some of the most complex information. As public information becomes more abundant, the challenge of making raw, unstructured data machine-readable arises. As a matter of fact, video and images are easier to comprehend than text. As an example, we can take "what's up." It is likely the human brain will interpret this phrase as a question, concern, or inquiry about someone. However, a machine may perceive the meaning of the text as it intends to, i.e., what is literally up, such as the fan, the ceiling, the ceiling.
Text annotations provide models with a better understanding of the data they are given, allowing them to interpret the text more accurately. During this session, we will cover some basic fundamentals of text annotation and how we at Cogito, as a leading data annotation company,, can help you with your annotation needs.
What is Text Annotation?
Labeling text documents or other content elements is a process called text annotation. Machines can sometimes be as intelligent as we are, but human language can be challenging to decrypt for machines unless they are trained with the right training data. As part of our text annotation services, we set some significant criteria to highlight specific sentence elements or structures to prepare training data for machines to recognize human language, intentions, and emotions.
Significance of Text Annotation for Machine Learning
What is the purpose of annotating text? Several breakthroughs in natural language processing (NLP) have shown that the demand for textual data is increasing across various industries, such as insurance, healthcare, banking, telecom, etc. Text annotation enables machine learning models to recognize the text contained in documents and the hidden sentiments within them.
The next section of this post will bring substantial insight into specific use cases of text annotation. For now, remember that text is still data and is manipulated similarly to images or videos for training and testing machines.
Annotating Text through Natural Language Processing (NLP)
There are many tasks that computers can now be taught to perform, but some activities remain untouched: Natural Language Processing (NLP) is one. In the absence of annotators, models cannot gain depth, naturalness, and in some cases, slang used in crafting, controlling, and manipulating language. It is for this reason that companies continue to turn to human annotators in order to ensure sufficient training data of the highest quality. NLP-based AI currently covers voice assistants, machine translation, chatbots, and alternative search engines, yet there is no end to the variety of text annotation types that can be used.
Text annotation for Optical character recognition (OCR)
Textual data is extracted from scanned documents or images (PDF, TIFF, JPG) using optical character recognition (OCR). Information can be made more accessible for users with OCR solutions. Managing unsearchable or hard-to-find data without the benefit of search optimizes business workflows and operations, saving time and resources. Using OCR-processed textual information makes it easier and faster for businesses to access and use information. Among the benefits of this technology are the elimination of manual data entry, the reduction of errors, and the improvement of productivity.
Types of Text Annotation
In text annotations, the text is underlined or highlighted, and margin notes are added. The main types of text annotations covered in this post are:
1. Entity Annotation
In chatbot training datasets, entity annotations are used to label unstructured sentences with important information. The following methods can be used to locate, extract, and tag entities in text:
Named Entity Recognition (NER): This technique is useful for locating people, geographic references, frequently occurring objects, and characters in the text. NER is fundamental to language processing. A few examples of NLP that use named entity recognition include Google Translate, Siri, and Grammarly.
Part-of-speech tagging: Part-of-speech tags are used to identify nouns, verbs, adjectives, pronouns, adverbs, prepositions, conjunctions, and more in sentences.
Keyphrase tagging: Identifying and labeling keywords in textual data can be accomplished using keyphrase tagging.
The entity annotation process involves combining entity recognition with part-of-speech and keyphrase recognition, and it often accompanies entity linking for a more complete contextualization.
2. Entity Linking
A named entity link (NEL), which is similar to entity annotation, connects these named entities with more comprehensive datasets. There is a difference between entity linking and NER. NER recognizes the named entity in the text without specifying which entity it denotes.
3. Text Classification
Text classification is the process of annotating chunks of text or lines with a single label instead of annotating individual words or phrases. Classifying documents, categorizing products, and annotating sentiments are examples of text classification.
Document classification: If there is a large amount of textual content in the document, assigning it a single label can aid in intuitive sorting.
Product categorization: It is possible to boost a product's visibility for an e-commerce website on the rankings page by categorizing or classifying products into classes and categories.
4. Sentiment Annotation
In general terms, sentiment annotation refers to identifying emotions, opinions, or sentiments within a text. Annotators analyze texts for emotions and opinions and select the label that best represents them. For instance, customer reviews could be analyzed. The annotations would be labeled as positive, neutral, or negative after the reviews were read.
Use cases of Text Annotation
Annotating text is almost as versatile as annotating images and videos. Different industries can use annotated textual data for model training in nearly every discipline. A number of industries have benefited from text annotation, including healthcare, banking, insurance, telecommunications, and others.
Automating heavy manual processes with high-performing models was possible through text annotation integration in AI-powered machines. In the not-too-distant future, there will be an increase in personalization, greater automation, reduced error rates, and adequate use of resources.
Final Thoughts
Text annotation can help the development of high-quality training data for NLP applications, such as chatbots, virtual assistants, search engines, and machine translation. We are happy to assist you with high-quality text annotations if you are planning to incorporate them into your machine learning initiatives. Cogito can offer a bouquet of text annotation services depending on your training data requirements. Originally published at Cogito Tech LLC
Comments