How Text Annotation Services Drive the Accuracy of Natural Language Processing Models
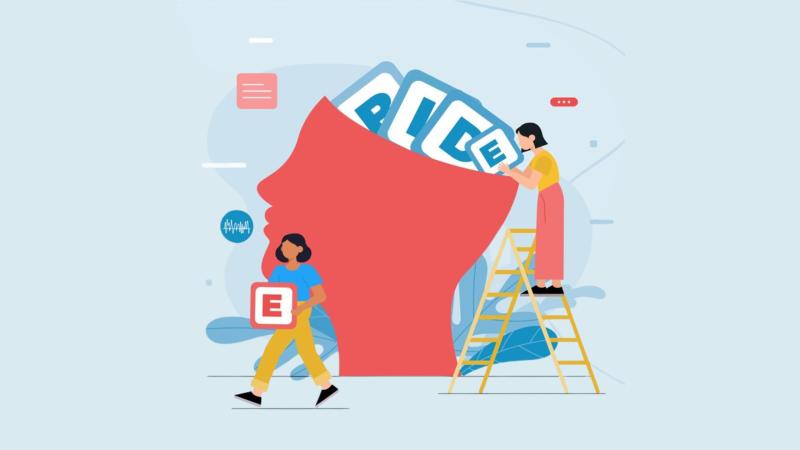
Ever wondered how virtual assistants like Siri and Alexa understand your commands and perform the desired actions? Or, what made Generative AI experiments like ChatGPT successful? Whether you want to summarize a long document, generate complex code, or write a simple blog, GPT can do it all! You simply need to give a few accurate prompts to get results within the snap of fingers.
These two are examples of natural language processing applications powered by text annotation. That's right! From voice assistants to chatbots and search engines to machine translation, NLP applications are powered by text annotation.
What is Text Annotation?
Do you think NLP models have brains? No, that’s not true! These applications need accurate, relevant, and high-quality text-based training datasets to understand their environment and perform the desired actions. That said, text annotation is the process of labeling textual data to make it machine-readable and suitable for training NLP models.
Elements like parts of speech, entities, sentiments, relationships, etc., within a dataset, are marked to help NLP algorithms understand and interpret human language. In essence, it bridges the gap between human language and machines, helping NLP models to comprehend raw text data and perform tasks such as classification, translation, and summarization.
Labeling customer feedback, for example, with sentiment categories as positive, negative, or neutral helps with sentiment analysis. Based on this, chatbots and virtual assistants identify the right mood and tone for conversation. Similarly, tagging entities like names, locations, or dates plays a key role in the development of applications like information retrieval systems and automated customer support. Isn't it interesting that machines understand your sentiments?
Role of Text Annotation in NLP Development
Going back in time, text annotation was merely limited to literature and academics, where people used to add notes to the written work. But now, it plays a key role in NLP model development, making language-based AI an integral part of our tech-driven lives. Think of the times when there were no voice assistants and chatbots!
At the same time, it is important to note that the quality and accuracy of data fed into NLP applications directly impact the output. Otherwise? The functionality of NLP models is limited, and they yield unreliable outputs. In short, text annotation ensures that data is easily comprehensible for ML algorithms and reduces the probability of biases affecting NLP applications by adhering to consistent guidelines.
And as NLP systems are applied across healthcare, finance, insurance, retail, and more industries, the need for accurate text annotation services becomes even more important. This is because any errors during the training and development process can put the entire model down in flames.
The Process of Text Annotation for NLP
Comments