The Role of Machine Learning in Advancing AI Applications
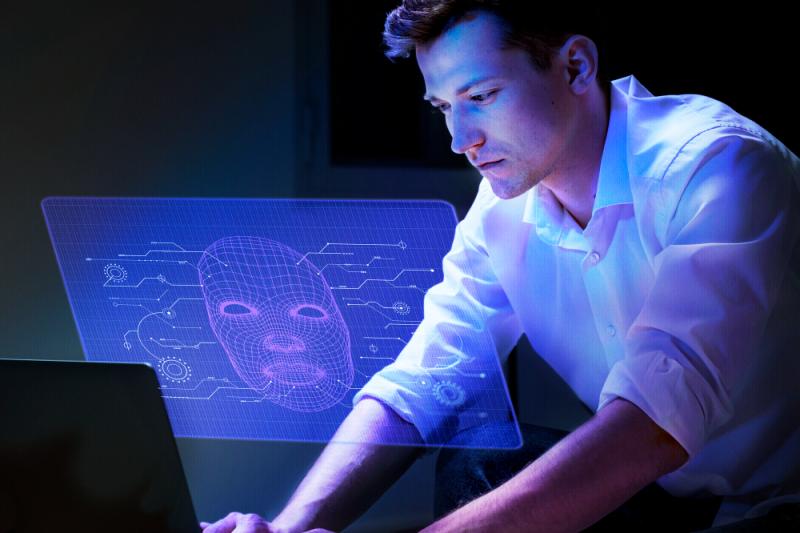
Artificial Intelligence (AI) has
become a cornerstone of modern technology, driving innovation across
industries. However, the real force behind many AI advancements lies in Machine
Learning (ML), a subset of AI that enables systems to learn and improve from
experience. Understanding the relationship between AI vs Machine Learning helps
us appreciate how these technologies complement each other to revolutionize
everything from healthcare to finance. Machine Learning serves as the engine
that powers AI, enabling it to solve complex problems, make predictions, and
perform tasks once thought exclusive to human intelligence.
How Machine Learning Drives AI Progress
1. Data-Driven Insights
Machine Learning relies on data
to identify patterns and make informed decisions. AI applications like
recommendation systems, fraud detection, and image recognition thrive on ML
algorithms that process vast amounts of data, turning raw information into actionable
insights.
2. Automation and Scalability
AI systems equipped with Machine
Learning can automate tasks at scale. For example, chatbots powered by Natural
Language Processing (a type of ML) handle customer queries efficiently, while
AI-driven supply chain systems optimize logistics and reduce costs.
3. Improved Accuracy Over Time
A key feature of Machine Learning
is its ability to improve with experience. Algorithms continuously refine their
predictions and decisions as more data becomes available. This iterative
learning process enhances the accuracy and reliability of AI applications over
time.
4. Expanding Use Cases
Machine Learning has opened the
door to applications once thought impossible. Self-driving cars, personalized
medicine, and real-time language translation are all powered by advanced ML
models that push the boundaries of AI.
Why Machine Learning is Indispensable for AI?
Machine Learning provides the
framework and algorithms that make AI adaptable and functional in real-world
scenarios. While AI defines the concept of machines simulating intelligence, ML
supplies the tools that enable these systems to learn and evolve. Without
machine learning, AI would remain static and unable to grow with changing data
or user needs.
Real-World Examples of ML Advancing AI
- Healthcare: AI systems use ML to analyze
medical images, diagnose diseases, and suggest personalized treatment
plans.
- Finance: Fraud detection algorithms rely on
Machine Learning to analyze transaction patterns and flag anomalies in
real-time.
- E-commerce: AI-powered recommendation
engines use ML to predict user preferences and enhance shopping
experiences.
Conclusion
The relationship between AI and
Machine Learning is not a rivalry but a synergy. Machine Learning is the
backbone of AI applications, enabling them to adapt, improve, and deliver value
across various domains. As technology advances, the partnership between AI and
ML will continue to redefine innovation, driving solutions that address today's
most complex challenges.
Comments (1)
Rajeev Patel
6
SEO Expert
Machine Learning Language is modern day programing language