How Machine Learning is Changing the Face of Predictive Analytics?
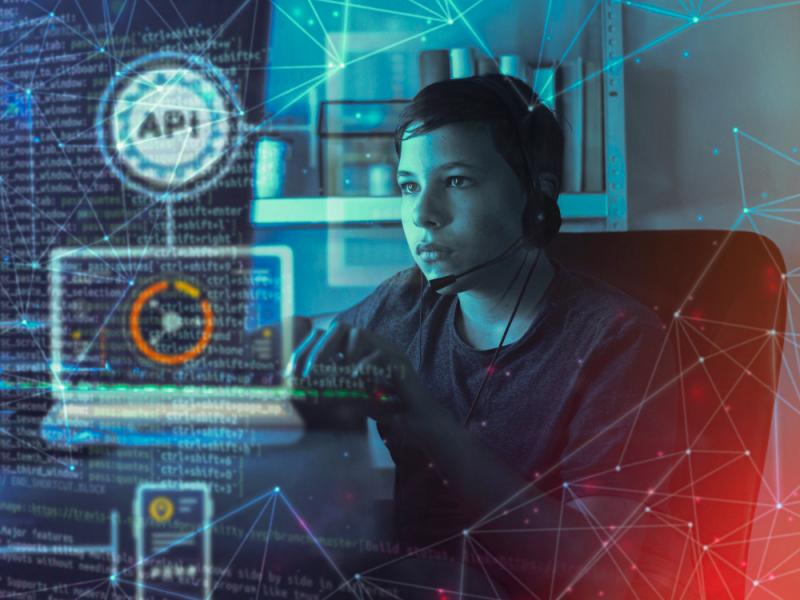
In today's data-driven world,
businesses rely on insights derived from vast amounts of data to make informed
decisions, forecast trends, and gain a competitive edge. Predictive analytics,
using historical data to predict future outcomes, has seen a radical
transformation thanks to the advances in machine learning technology. With the
support of machine learning development services, organizations can now build
robust, accurate models that improve predictive capabilities and enable highly
personalized experiences and proactive decision-making.
The Power of Machine Learning in Predictive
Analytics
Traditional predictive models
were often based on linear relationships and rule-based algorithms requiring
human intervention and limited flexibility. However, With machine learning,
predictive analytics can leverage vast datasets, identify complex patterns, and
self-improve over time. Machine learning models can automatically learn from
new data, uncover hidden relationships, and adapt to changing conditions,
making predictions more reliable and relevant.
For example, e-commerce and
digital marketing businesses use machine learning to analyze customer behavior
and predict future purchasing trends. Financial institutions use it to forecast
stock market trends and detect fraud, while healthcare providers employ it to
anticipate patient health risks. Machine learning increasingly enables
predictive analytics to be more accurate, efficient, and impactful.
Key Machine Learning Techniques Powering
Predictive Analytics
1. Supervised Learning:
Supervised learning is widely
used in predictive analytics to train models on labeled datasets. This
technique helps in making accurate predictions based on historical data. For
instance, a model trained with transaction data can identify patterns to predict
future transactions or flag potential fraud.
2. Unsupervised Learning:
Unsupervised learning is used
when the data lacks labeled outputs, making it helpful in discovering hidden
patterns. In predictive analytics, clustering techniques in unsupervised
learning help segment customer data for better-targeted marketing.
3. Deep Learning:
Deep learning, a subset of
machine learning, is particularly effective in predictive analytics for
handling large, unstructured datasets such as images and text. This technique
is highly relevant in fields like healthcare, where deep learning models can analyze
medical records to predict patient outcomes or detect early signs of diseases.
4. Reinforcement Learning:
Although less common in
traditional predictive analytics, reinforcement learning is emerging as a
valuable technique for applications that require dynamic decision-making, such
as supply chain optimization and personalized recommendations in real time.
Applications of Machine Learning in
Predictive Analytics
Machine learning has opened
endless possibilities across various sectors for predictive analytics:
1. Customer Behavior Prediction:
Companies can predict future
buying patterns by analyzing customer interactions, purchase history, and
browsing behavior and adjust marketing strategies to maximize engagement and
conversions.
2. Predictive Maintenance in Manufacturing:
Machine learning algorithms can
analyze machinery data to predict when equipment will likely fail, allowing
companies to schedule timely maintenance and avoid costly downtimes.
3. Fraud Detection in Financial Services:
In the financial industry,
machine learning models detect real-time anomalies, which helps identify and
prevent transactions before they occur.
4. Healthcare Predictive Models:
Predictive analytics in
healthcare can analyze patient data to forecast health risks, improve patient
outcomes, and assist in developing personalized treatment plans.
5. Supply Chain and Inventory Management:
Machine learning models help
businesses anticipate demand fluctuations, optimize inventory, and streamline
supply chain operations by predicting stock needs based on past patterns and
market conditions.
Benefits of Machine Learning in Predictive
Analytics
1. Enhanced Accuracy and Efficiency:
Machine learning models
continuously learn from data, refining their predictive capabilities and
improving accuracy.
2. Scalability with Big Data:
Machine learning is well-suited
for large datasets, enabling predictive analytics to handle previously
unmanageable data with traditional methods.
3. Real-time Insights and Responsiveness:
Unlike traditional analytics,
machine learning-powered predictive models can process data in real time,
allowing organizations to make immediate adjustments and respond proactively.
4. Reduction of Human Bias:
Machine learning reduces the
impact of human bias in data analysis by relying on objective algorithms, which
ensures a more accurate representation of patterns and predictions.
Challenges and Considerations in Implementing
Machine Learning for Predictive Analytics
Despite its transformative
potential, implementing machine learning in predictive analytics comes with
challenges:
1. Data Quality and Availability:
Machine learning relies heavily
on high-quality data for accurate predictions. Data preprocessing and cleaning
are essential to remove inconsistencies.
2. Model Interpretability:
Many machine learning models,
such as intense learning, function as "black boxes," making it
difficult to interpret how confident predictions are made.
3. Ethical and Privacy Concerns:
As machine learning models
analyze personal data for predictive purposes, handling data responsibly and
complying with regulations like GDPR to protect user privacy is critical.
4. Skilled Talent Requirement:
Leveraging machine learning for
predictive analytics requires a specialized skill set, including data science
and machine learning expertise.
How Machine Learning Development Services
Facilitate Predictive Analytics?
Partnering with a Machine
Learning Development Services provider can simplify the implementation process.
These providers offer expertise in data preparation, model selection, and
deployment strategies tailored to specific business needs. They ensure that
predictive models are designed to handle the complexities of real-world data
and align with the organization's objectives, enabling faster and more
effective results.
Conclusion
As machine learning continues to
evolve, its role in predictive analytics will only grow stronger. Advancements
in algorithms increased computational power, and access to big data is expected
to make predictive models even more accurate and adaptable. We may soon see
predictive analytics used to make real-time adjustments in everything from
marketing campaigns to healthcare treatments.
Machine learning is undoubtedly
changing the face of predictive analytics by making it brighter, faster, and
more powerful. With continued advancements and the support of expert Machine
Learning Development Services, businesses will be well-positioned to harness
predictive analytics as a core component of their decision-making processes,
driving unprecedented levels of accuracy and efficiency.
Comments