From Classical to Generative AI: Understanding the Evolution of AI Technologies
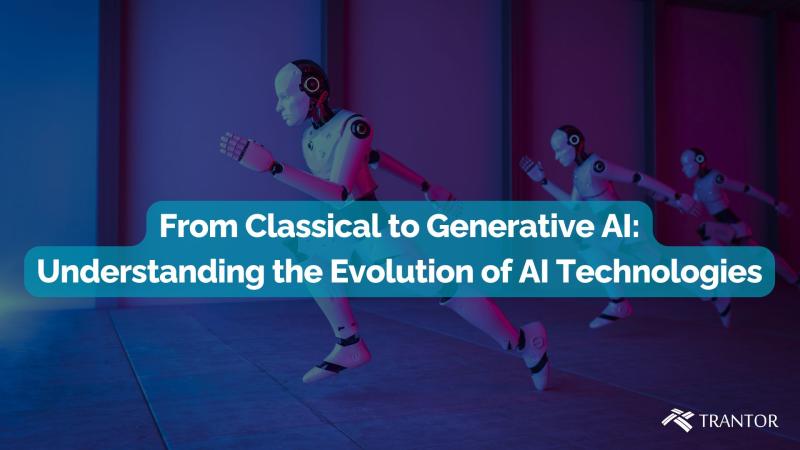
Artificial Intelligence (AI) has transformed industries and redefined how businesses and individuals interact with technology. Over the decades, AI has evolved dramatically, transitioning from simple rule-based systems, often referred to as classical AI, to complex and creative generative AI. As businesses adapt to the changing digital landscape, it’s essential to understand the fundamental shifts in AI technologies and their implications for enterprise solutions, strategy, and innovation.
In this blog, we will explore the evolution of AI from classical systems to the cutting-edge world of generative AI, covering its defining characteristics, differences, and use cases. This journey not only highlights the growth of AI but also emphasizes the importance of selecting the right AI technologies to drive business transformation.
The Era of Classical AI: Rule-Based Systems
Classical AI, also referred to as "Good Old-Fashioned Artificial Intelligence" (GOFAI), was built on logic, rule-based systems, and decision trees. The main approach here was deterministic: AI was programmed to follow specific rules to achieve pre-defined objectives.
Key Features of Classical AI:
- Rule-Based Systems: At its core, classical AI operates based on predefined rules and algorithms. These systems are designed to solve problems within a constrained environment, where every potential scenario is accounted for in the programming.
- Symbolic AI: Classical AI relied on symbolic representations, where variables, objects, and conditions were encoded in logic. This allowed for easy understanding and manipulation by computers.
- Fixed Operations: Classical AI could only perform tasks based on the specific instructions given to it. It lacked the flexibility to adapt or generate new solutions beyond its programming.
Use Cases of Classical AI:
- Expert Systems: Classical AI was widely used in early expert systems, where the goal was to replicate human expertise in specialized domains like medical diagnosis or financial analysis.
- Chess Algorithms: AI-based chess programs such as IBM’s Deep Blue leveraged rule-based systems to evaluate possible moves and compute the best outcomes.
- Early Search Engines: Search engines in the early days relied heavily on classical AI to index and retrieve web content based on keyword matching.
Classical AI helped businesses automate routine tasks, but the rigidity of rule-based systems limited their scope. The major challenge was scalability — when environments grew more complex, the limitations of classical AI became more evident.
The Rise of Machine Learning and Neural Networks
As computing power increased and datasets became larger, AI researchers sought more adaptive and scalable solutions. This led to the rise of machine learning (ML) and neural networks in the late 20th century. Instead of relying solely on rule-based systems, these technologies enabled AI to learn from data and improve over time.
Key Innovations in Machine Learning:
- Data-Driven Approach: Machine learning focuses on creating models that can identify patterns and make decisions based on input data. The more data the model is exposed to, the better it becomes at making predictions.
- Supervised and Unsupervised Learning: Supervised learning allows models to be trained using labeled datasets, while unsupervised learning can discover patterns in unlabelled data. Both approaches improved AI's adaptability across different domains.
- Deep Learning and Neural Networks: The advent of deep learning revolutionized AI with multilayered neural networks that mimic the human brain’s functioning. These networks excel at image recognition, natural language processing (NLP), and other complex tasks.
Machine learning drastically improved AI’s ability to handle vast and diverse data streams, enabling systems to predict outcomes, detect anomalies, and uncover hidden insights. As this phase of AI evolved, it laid the groundwork for more sophisticated developments in AI — particularly generative AI.
Generative AI: The New Frontier
Generative AI represents the next significant leap in artificial intelligence. Unlike classical AI, which relies on predefined rules, or even machine learning, which uses data to make predictions, generative AI creates entirely new content based on input data. This opens up unprecedented possibilities for creativity, innovation, and automation.
What is Generative AI? Generative AI refers to AI systems that can generate new data, such as text, images, videos, or even music, by learning patterns from large datasets. These systems use advanced models such as Generative Adversarial Networks (GANs) and transformer-based models like GPT (Generative Pre-trained Transformer).
Key Features of Generative AI:
- Content Creation: Generative AI can produce entirely new outputs, such as writing human-like text, creating artwork, or composing music, without needing explicit programming for each scenario.
- Self-Learning Models: Unlike classical AI, generative AI models continue to improve their ability to generate creative outputs by iterating on the data they’re trained on.
- Natural Language Understanding: Generative AI excels at natural language processing (NLP), allowing machines to understand and respond to human speech more fluently and coherently.
Generative AI vs Traditional AI
While traditional AI (whether rule-based or predictive) focuses on analyzing data to predict outcomes, generative AI creates new data by learning the underlying structure of its training data. This shift from data analysis to data generation represents a fundamental change in AI’s capabilities. Generative AI vs Traditional AI comparisons highlight how generative models offer more flexibility, adaptability, and creative potential than their classical or predictive counterparts.
Use Cases of Generative AI
Generative AI is quickly transforming industries and reshaping how businesses approach content creation, product development, and customer interaction.
- Content Generation:
- Tools like OpenAI’s GPT models are revolutionizing content creation by automating the generation of articles, blog posts, and social media content. These models can write compelling, human-like text based on simple prompts, streamlining the content production process for marketing and media companies.
- Image and Video Synthesis:
- GANs have been used to create hyper-realistic images, videos, and animations. Industries such as advertising, entertainment, and gaming are leveraging these models to create visual content at scale without manual effort.
- Healthcare:
- Generative AI is helping medical researchers simulate complex biological processes, design new drugs, and generate personalized treatment plans by analyzing patient data and predicting medical outcomes.
- Product Design:
- AI can now design new products and suggest innovative improvements to existing ones by analyzing market data, customer preferences, and design trends.
- Virtual Assistants:
- Virtual assistants and chatbots powered by generative AI models offer more engaging and personalized user interactions. These assistants are capable of understanding context, generating meaningful responses, and improving user experiences over time.
The Future of AI: Beyond Generative Capabilities
As generative AI continues to mature, it’s likely to be integrated with other advanced technologies like reinforcement learning, edge computing, and quantum computing. These integrations could lead to the development of more autonomous AI systems that can make high-level decisions, manage businesses, and even interact in social environments.
For businesses like Trantor, investing in generative AI can offer significant competitive advantages. By deploying AI solutions tailored to specific business needs, companies can enhance efficiency, improve customer engagement, and streamline operations in ways that were unimaginable with traditional AI. Trantor’s expertise in generative AI solutions is already helping clients navigate the AI revolution, making cutting-edge AI accessible to enterprises of all sizes.
Conclusion
The evolution of AI technologies from classical, rule-based systems to advanced generative models reflects a paradigm shift in how machines interact with data, humans, and the environment. Classical AI laid the foundation for modern AI, but the emergence of generative AI is unlocking new possibilities for creativity, innovation, and automation across industries.
As businesses look ahead, understanding the differences between generative AI vs traditional AI is crucial to making informed decisions about which technologies to adopt. Whether you're focused on predictive analytics or looking to harness the power of generative models, AI is no longer a distant concept—it’s a tool that is reshaping the future of enterprise solutions.
For enterprises ready to explore the potential of generative AI, companies like Trantor provide consulting, implementation, and strategy services to help leverage these advanced technologies for business growth. By understanding the evolution of AI, businesses can position themselves at the forefront of digital innovation.
Comments