Understanding Data Lakes: The Future of Data Storage and Analysis
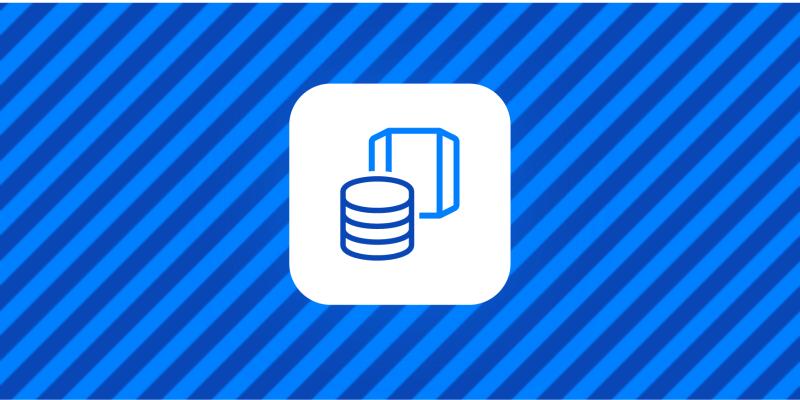
In today’s data-driven world, organizations are collecting vast amounts of data from various sources, including social media, IoT devices, and transactional systems. To harness the potential of this data, businesses are increasingly turning to data lakes as a solution for storage and analysis. But what exactly is a data lake, and how does it differ from traditional data storage methods? Let’s delve into the concept of data lakes, their benefits, and their role in modern data strategies.
What is a Data Lake?
A data lake is a centralized repository that allows you to store all your structured and unstructured data at any scale. Unlike traditional data warehouses, which store data in predefined schemas and formats, data lakes are designed to hold raw data in its native format until it is needed for analysis. This means that you can store everything from relational data to binary data, such as images, videos, and logs, all in one place.
Data lakes leverage technologies like Hadoop and cloud storage solutions (e.g., Amazon S3, Azure Data Lake Storage) to provide a scalable, cost-effective way to store and manage large volumes of data.
Key Characteristics of Data Lakes
Schema-on-Read: Data lakes services utilize a schema-on-read approach, meaning that the structure of the data is determined when it is read or processed, rather than when it is stored. This flexibility allows organizations to adapt to changing data requirements quickly.
Storage of Diverse Data Types: Data lakes can store various data types, including structured, semi-structured, and unstructured data. This capability enables organizations to maintain a comprehensive dataset for analytics and machine learning.
Scalability: Built on distributed systems, data lakes can scale horizontally to accommodate growing data volumes without significant infrastructure changes.
Cost-Effectiveness: By utilizing commodity hardware and cloud-based storage solutions, data lakes provide a cost-effective option for storing large amounts of data.
Benefits of Using Data Lakes
Enhanced Data Accessibility: Data lakes facilitate easier access to a broader range of data, empowering data scientists and analysts to work with various datasets to derive insights and make informed decisions.
Fostering Innovation: With the ability to experiment with different data types and analytics models, organizations can drive innovation and explore new business opportunities.
Support for Advanced Analytics: Data lakes provide the foundation for advanced analytics, including machine learning and AI, enabling organizations to leverage predictive modeling and real-time analytics.
Improved Collaboration: By centralizing data storage, data lakes promote collaboration across teams, allowing data engineers, analysts, and data scientists to work together more effectively.
Data Lakes vs. Data Warehouses
While both data lakes and data warehouses serve the purpose of data storage and analysis, they cater to different needs:
Structure: Data warehouses use a schema-on-write approach, requiring data to be structured before storage. In contrast, data lakes use a schema-on-read approach, allowing raw data to be stored until needed.
Data Types: Data warehouses primarily handle structured data, while data lakes can accommodate structured, semi-structured, and unstructured data.
Use Cases: Data warehouses are ideal for business intelligence and reporting, whereas data lakes are suited for big data analytics, machine learning, and exploratory data analysis.
Best Practices for Implementing a Data Lake
Define Clear Objectives: Before building a data lake, organizations should define clear objectives and use cases to guide the design and implementation.
Implement Data Governance: Establishing data governance practices is crucial to ensure data quality, security, and compliance within the data lake.
Utilize Metadata Management: Effective metadata management helps in organizing and managing the data stored in the lake, making it easier to locate and use.
Leverage the Right Tools: Choose the right data processing and analytics tools that can effectively integrate with your data lake architecture.
Conclusion
Data lakes are revolutionizing the way organizations store, manage, and analyze data. By providing a flexible, scalable, and cost-effective solution for handling diverse data types, data lakes empower businesses to unlock the full potential of their data. As organizations continue to embrace data-driven strategies, understanding and implementing data lakes will be crucial for achieving competitive advantages in today’s fast-paced digital landscape.
Comments