Evaluating SaaS AI Demand Forecasting Performance
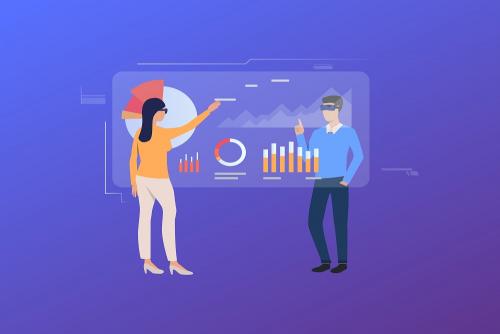
At the current pace of change in the world of business it is impossible not to forecast demand precisely. It is used by businesses in various fields to decide on the right quantity to buy and the right amount to produce to achieve a higher level of profitability. Such traditional forecasting techniques may be inadequate because they fail to explain changes in demand based on the degrees of internal and external forces that impact these changes. Here comes the new driving force – SaaS AI demand forecasting – the right combination of artificial intelligence predictors and cloud technologies to revolutionize forecasting.
So, how do you determine the usability of a SaaS AI demand forecasting solution? This article goes into the depth analysis of the evaluation for your business and all of it should help you better understand the evaluation process for your business.
Demand Forecasting for SaaS AI
AI demand forecasting solutions for SaaS are software applications that belong to the cloud computing sector that are built on data sets and rely on big data algorithms. This data could range from internal – historical sales reports in a company, POS data, demographics information about the customers, external – information about the weather, economic trends, and social media sentiment. With these complex pieces of information, the AI models are able to detect patterns that are not easily observable and show seasonality in the demand that is ignored by any ordinary methods.
The core strength of SaaS AI demand forecasting lies in its ability to:
Handle non-linear relationships: Traditional approaches tend to work with a high level of assumption that variables are linearly related. On the other hand, AI is able to reveal nonlinear and complicated trends in the data, which results in more accurate predictions.
Learn and adapt dynamically: AI models like ClearVista constantly learn and update their predictions based on the new data. This makes it easier for you to make future predictions that are relevant to current market trends.
Integrate with existing systems: It, however, has the capability of easily integrating with enterprise resource planning (ERP), and customer relationship management (CRM) as well as providing for easy and smooth transition in the forecasting systems and processes.
SaaS AI Demand Forecasting Performance Analytics & KPIs.
Before or while planning on implementing a SaaS AI demand forecasting system, it is important that KPIs are identified to easily quantify the ability of the system to perform effectively. Some critical metrics to consider:
Forecast Accuracy: This is one of the basic indicators. It is the measure of the accuracy of the forecast, the extent to which a forecast is close to actual demand. MAE, MSE, or MFE may be added to be used as the accuracy measures.
Forecast Bias: This refers to the sympathy situation in which the demand is inflated or deflated by the forecast. Bias testing makes it possible to determine whether the model has any inclinations to the outputs.
Forecast Rolling Average (FRA): This is a measurement of the forecast error of a statement within a particular period (weekly, monthly). Reviewing the FRA provides a means of pinpointing times in which progress in the forecast prediction quality has been incrementing or diminishing.
Inventory Levels: When a SaaS AI demand forecasting platform is working effectively this process should bring about improved orders and inventory. Check stock-keeping in order to identify whether there are stock shortages and overstocking illnesses.
Service Levels: It is believed that the forecasting tool should help you release the right quantities to fulfill customer expectations. Track service levels (e. g. The effectiveness and efficiency of the service quality (on-time deliveries, addressing customers’ needs and expectations) to reach a high level of customer satisfaction.
Conducting a Pilot Test
Bringing on board a new SaaS AI demand forecasting system should not be done in a general manner. It helps in software testing and assessing the efficacy of the software for the specific task and your data.
Data Preparation: Take time while preparing your historical data to be cleaned and accurate as well as ensure it is in a format that will work for the SaaS AI platform of interest.
Model Configuration: Help the solution provider to customize the AI model to the specific circumstances of your industry or product categories and the kinds of forecasts you need to make.
Forecast Generation: Implement the pilot on a partially- or fully-depleted data set to check the performance of produced predictions.
Performance Evaluation: Assess actual demand data against the forecasted inventory levels produced during the pilot using the aforementioned KPIs.
Feedback and Refinement: Deliver the results of the pilot back to the solution provider and give him or her recommendations. This assures that some corrections and modifications are achieved on the model before full deployment can be done.
The pilot test is about testing the SaaS AI demand forecasting solution before use and gathering information about the actual capacity of the SaaS AI demand forecasting solution to determine whether it is a good fit for your business.
Other Aspects of Evaluation
Beyond the technical aspects, there are several other points to consider when evaluating a SaaS AI demand forecasting solution:
Ease of Use: The platform should be well-designed with self-explanatory and layman-friendly interfaces to make it easy for your team to understand, read, and use the generated forecasts.
Scalability: Pick a plan that will work for a larger company to plan for the future.
Data Security: Those are the areas that answer the question of why the chosen platform would suffice since the solution entails having information that is highly confidential to the business.
Vendor Support: Correct selection of the SaaS AI demand forecasting support vendors that will be involved in the SaaS AI demand forecasting solution implementation and maintenance is also important. Assess the vendor in terms of its past performance when it comes to timely and effective support.
Performance Comparison of AI-based SaaS Platforms for Demand Forecasting
To choose the right SaaS AI demand forecasting solutions after reviewing some candidates, benchmarking can assist the user in making an informed decision.
Identify Competitors: Remember the approaches adopted by companies with the same or similar corporate strategy.
Gather Information: Continue researching online reviews, case studies, and industry reports to identify the capabilities of other solutions in terms of their accuracy, ease of use, and their ability to generate a return on investment.
Request Demos: Organise product demonstrations with those suppliers that passed through to have a practical feel of the platform. This makes it possible to view the solution closely and ask any specific questions that are subject to personal needs.
Compare Features: Make a comparison matrix to document main differentiation factors such as supported forecasting techniques, visualization abilities, and report generation.
Cost Analysis: Determine how much it will be to acquire the hotel for that price. Take into account the underlying cost of ownership, which may include subscription fees for a given period, as well as fees for implementation and training.
Benchmarking key factors will give you an overview of the SaaS AI demand forecasting services you can get and the strengths and weaknesses highlighted by each service provider.
Human Capital and SaaS AI Demand Forecasting
This is where AI demand forecasting in the SaaS AI space is key, yet the human element always remains essential.
Domain Expertise: Follow the recommendations of the sales and marketing people when it comes to learning the AI model and interpreting things that have been predicted by the AI model.
Data Quality Management: Have an established data governance system to maintain the quality of data used to feed to the AI model.
Scenario Planning: forecasting models should be used as a reference but not a source of reliance. Forecast with human expertise to anticipate unaccounted events and adjust accordingly.
Based on the situation in the market of the SaaS AI demand forecasting and use it in the highly competitive environment, the author advocates the possible synergy of artificial intelligence and the human will as the best collaboration in actualizing it.
Conclusion
Being able to specify important features and find improvement metrics that reflect the increase in your solution’s predictive performance for addressing whether your solution is effective in increasing the accuracy of your financial predictions for expressing whether a business succeeds or fails, you can do additional pilot tests to check the effectiveness of your solution.
In summary, technology is simply a factor that will need to be used in conjunction with data governance, human resources, and participant orientation to ensure that implementation is done right. We have presented the evaluation process as an effective tool for measuring how well an organization is managed and how the application of the power of combined human AI talents can help one achieve the desired changes.
Comments